
Director of Sales
Understanding Web3 Security’s Best Countermeasure: Machine Learning
Recently, a prominent exchange listed a job posting for: Fraud Analyst (24/7 schedule)
That’s exactly the title of the role.
A couple of the job tasks included:
- Perform ongoing monitoring of customer behavior and proactively detect new fraud patterns and suspicious tendencies
- Monitor and analyze the potential risk of fraud through holistic reviews and data analysis
- Regularly review the effectiveness of fraud rules and optimize them to improve accuracy
24/7. Optimizing. Analyzing. Monitoring. Whew – exhausting.
It’s time to talk about machine learning. One of the features of blockchains, is data. One of the bugs of crypto is fraud.
The combination creates a perfect opportunity for applying machine learning.
Clarifying Machine Learning
First off, we’re not quite talking about AI. While we’ve mentioned the FTE above, it’s still important blockchain companies deploy the right human resources for taking action on what’s learned from the data.
Artificial Intelligence vs Machine Learning
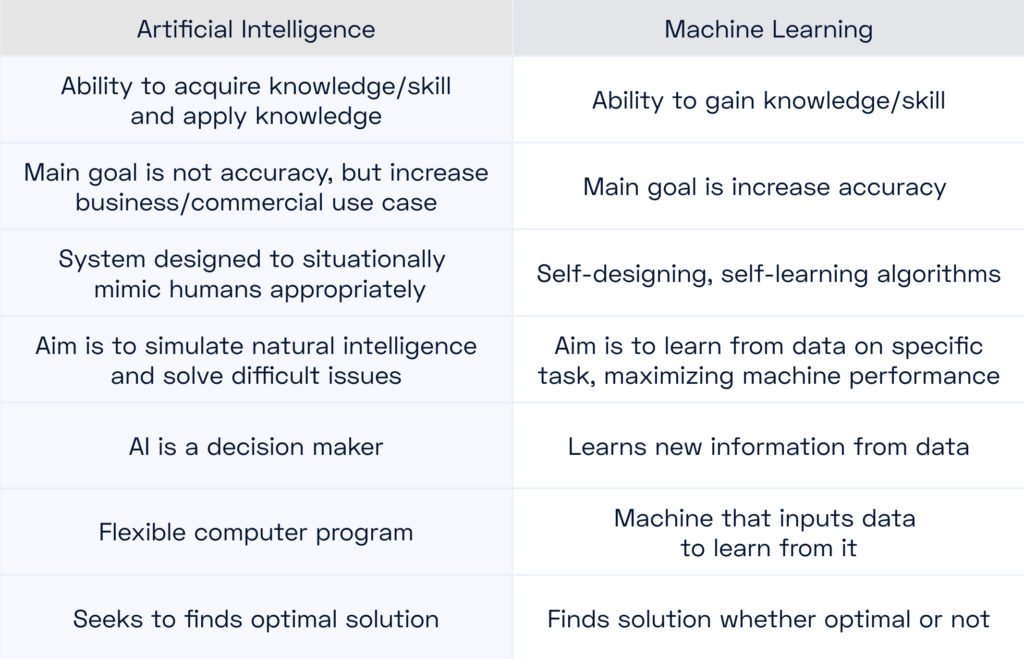
For Fraud detection, we aspire to high accuracy, focus on a specific task, learn new things from the data (emerging threat vectors), and benefit from the abundance of data that blockchain and elsewhere can supply. Naturally, machine learning fits the bill.
Secondly, machine learning and heuristic modeling solve different problems. Heuristics is pattern matching (solves a purpose), ML is training models (thousands of data points to identify patterns).
For example, at CUBE3.AI, we first use heuristics to clean data prior to feeding models, which is an important step in enabling highly efficacious ML.
Heuristics solve simple problems. ML solves complex problems. On-chain fraud detection is a complex problem.
Applying Machine Learning to Fraud Detection
Fraud in web3 tends to follow a similar pattern and timeline of events as we’ve outlined in previous a post.
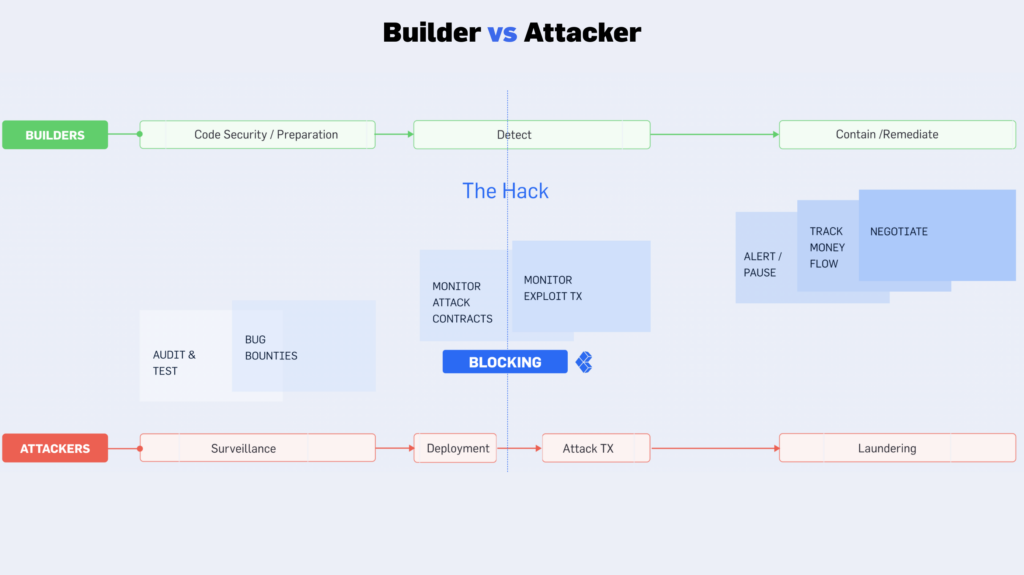
Simply from the graphic, the attacker determines the vulnerability they’ll exploit, builds the malicious strategy, deploys the smart contract that will be used for the exploit, funds that contract, and then performs the attack.
Once the attack transaction is live, it’s too late to prevent and victims are only left with the option of deploying remediation efforts.
So when machine learning is leveraged, it can directly impact the outcome.
There’s little that can be done at the stage the attacker is surveilling their exploit opportunities.
Catching threats in the mempool is inherently a risky proposition. One example: “25% of the hacks found end up being blocked.”
In security, leaving things to a level of chance helps no one sleep at night. This is not to mention, it’s extremely expensive to attempt front running and no one wants to burn money.
Ideally, the when, is before the attack transaction.
At CUBE3.AI, we decided at the point an attacker deploys their attack contract is the right time to leverage machine learning.
How Does Machine Learning Web3 Security Work?
Machine Learning allows us to identify contracts that are meant for malicious purposes. We recognize this within seconds after the deployment. Our risk scoring evolves and is improved as attacks add confirmation to our conclusions. The data we create is baked back into our modeling refinement.
This threat detection enables automation in replacement of manual response.
There’s a limited amount that can be shared here. If you’d like to know more about how it works –
The Benefits When Done Right
Foundations are key in this topic.
When in the data, you begin the ML and the precision of your models are critical.
Secondly is efficacy, and there are two things to keep top of mind:
- Accuracy determines your false positive rate, which can cause a few sneaky downstream issues. For starters, it’s simple, if you get it wrong you’ll trigger the wrong (often unwanted) action. But if you take a closer look, you’ll find even deeper issues. One example, if your models throw off too many incorrect outputs your interest and focus on those outputs naturally begins to wane. It’s a mirror of the childhood story “The Boy Who Cried Wolf” and as you’d expect, teams leveraging low efficacy modeling will slowly become more and more susceptible to attacks. If this happens, you may end up worse than when you started.
- High efficacy breeds improved data to feed your models and enables massive downstream product breakthroughs. You can envision benefits like scaling automation for labeling (explainability) and exponential improvement in screening out FPRs.
ML is only as good as your training data.
At CUBE3.AI, we acknowledge:
- Public labeled data sets have a high false positive rate – sometimes 40-50%.
- It takes a LOT of work to prep the data. Like mentioned before, we meticulously clean our training data via heuristics.
- An accurate way to test and qualify models is necessary, requires large human resources, and is constantly ongoing.
The right foundation is highly efficacious ML smart contract detection. The benefits will be experienced in short term breakthroughs and long term exceptionality in the models.
Web3 Machine Learning Security Use Cases
Blocking Crime Before it Happens
We live in an era where billions of dollars are lost from web3 hacks every year. It’s also a time when the technology to prevent this from happening exists. The question now is will teams take the action to deploy the measures needed for solving this problem.
Web3 uniquely presents the feature where transactions cannot be reversed, and therefore an issue when hacks can not be reversed.
It also presents an abundance of data that can be acted upon for developing precise intelligence for preventing attacks.
Best in class machine learning will make the most meaningful step in combating web3 security threats and enable stopping them before they happen.
Decreasing Time to Response
While smart contract owners will make decisions on preventative automation, 3rd parties will need to identify means for responding to threats, in-real time. For example, investors LPing on a protocol care less about protecting that protocol from attacks themselves. They do, however, care greatly about being the first to react when things go wrong. This is a competitive advantage and a risk mechanism for managing their downside. ML will increase this advantage via higher speed and earlier detection.
Accelerating Development of Security User Products
Coinbase is making massive strides in this area. Fast-forward to the 6:06 mark in this presentation, where you’ll find the Coinbase team using Machine Learning to build:
- Alerts for users during transactions
- Capabilities for hiding scam tokens from users in their web3 wallet
- Intelligence for surveilling stolen funds from banks to their exchange and then elsewhere
- Much more…
Ensuring Regulatory Compliance
2024 has already presented a strong trend of increased regulatory progress, which we have previously written about here. Most notably, the SEC has approved BTC ETFs.
The EU is also marching towards sweeping changes to regulation not only impacting CeFi but also DeFi.
- MiCA, EU’s Comprehensive New Crypto Regulation, Explained
- EU comes to provisional agreement to expand AML to crypto
- Crypto Compliance in 2024
The combination of ML powered Cyber/Fraud detection AND Compliance data will uplevel the industry’s ability to prepare and comply with upcoming regulation that will benefit all of our web3 objectives.
Conclusions on Machine Learning Web3 Security
The potential of web3 is why we’re all here. Incrementally we’re stepping closer and closer to this future reality yet the speed of which we realize this opportunity will never be fast enough. Securing our industry today will protect our collective future opportunity and accelerate the time it takes to get there.
Machine Learning will play a critical role, and CUBE3.AI is here to innovate and harness the tools to make web3 safer for our clients and more secure for the entire community.
Contact us, review our docs and access our free tools by signing up and joining our community today!